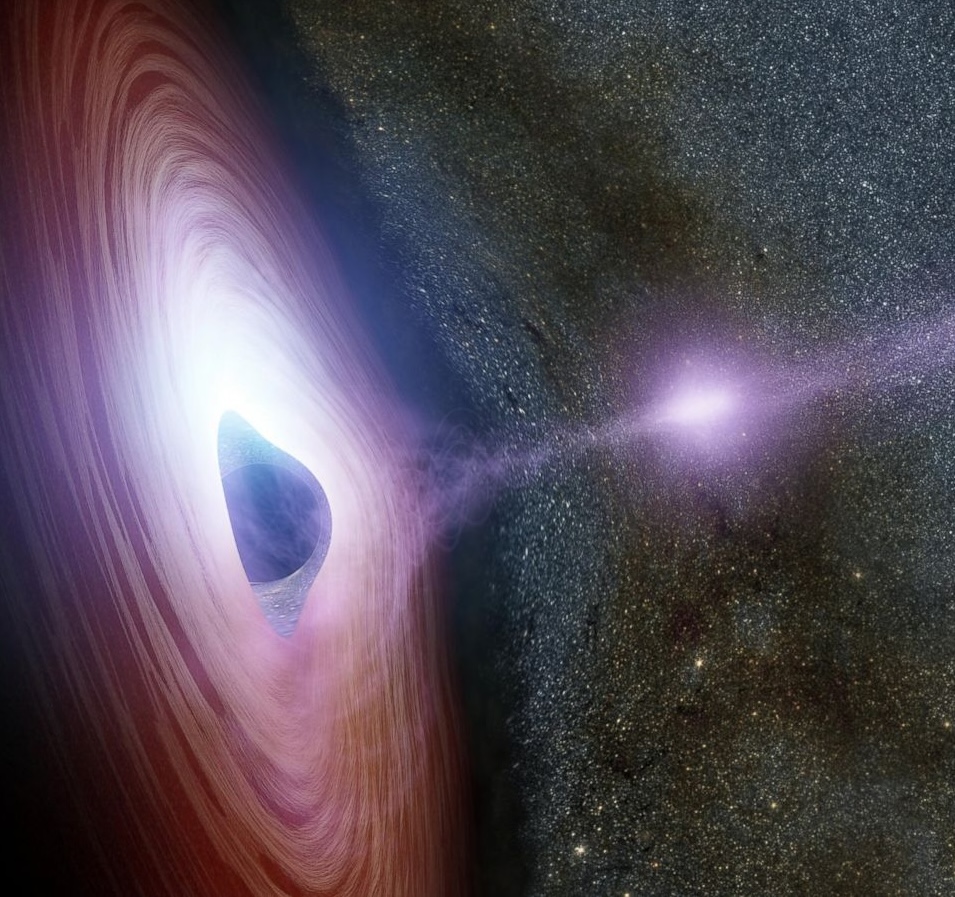
Hunting the Most Massive Black Holes
Our research is dedicated to identifying and studying the most massive black holes in the Universe. These giants, often located in the centers of galaxy clusters, provide a unique opportunity to explore how black holes shape their surroundings and influence the evolution of galaxies. By using a combination of multi-wavelength observations and advanced techniques, including machine learning, we aim to uncover how these black holes regulate gas flows, drive galaxy transformations, and affect large-scale cosmic structures. Through this work, we hope to deepen our understanding of the profound impact these extraordinary objects have on the Universe.
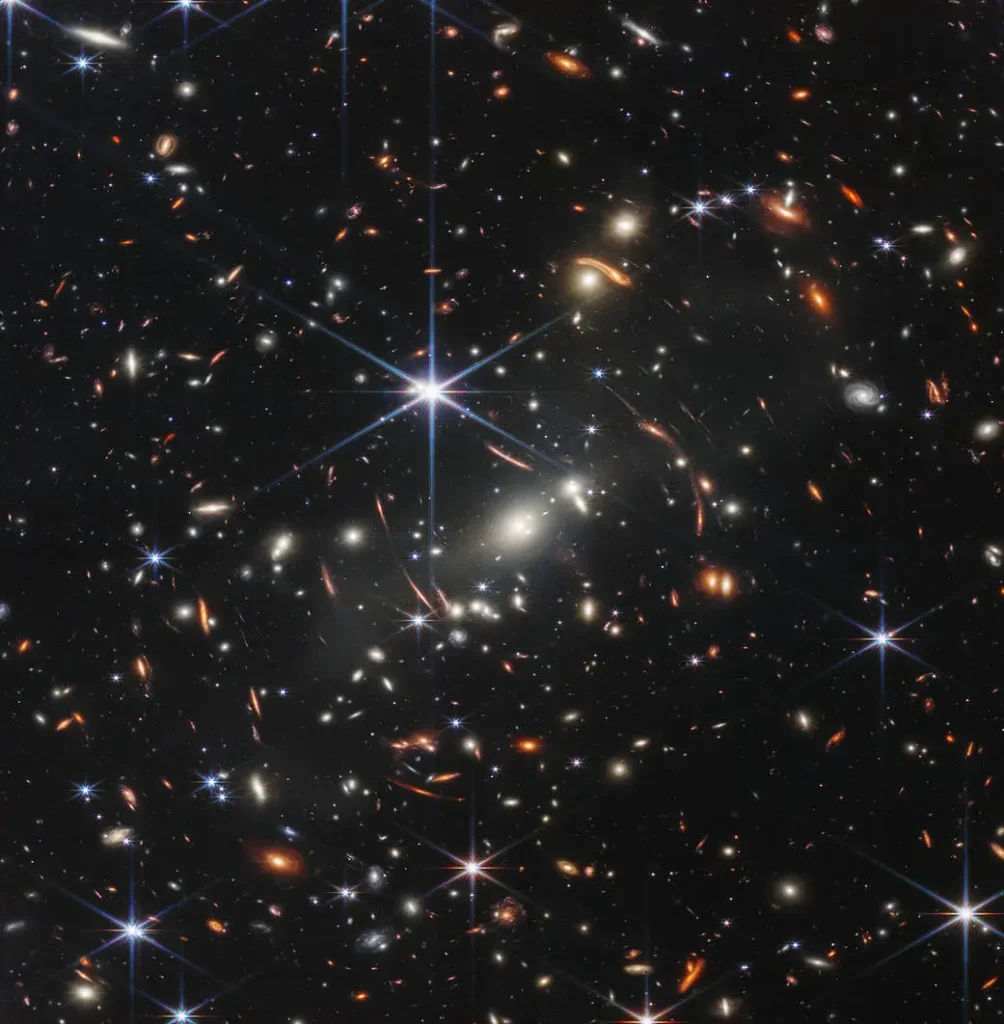
Clusters of Galaxies: Exploring High-Redshift Frontiers
Our team investigates galaxy clusters, the largest gravitationally bound structures in the Universe, with a focus on high-redshift clusters. By examining the role of black holes in these distant systems, we aim to understand how they influence galaxy evolution over cosmic timescales. Using advanced X-ray and radio observations, such as Chandra, XMM-Newton, JVLA and LOFAR, we are uncovering the intricate interplay between black holes and their environments, contributing to a better understanding of the evolution of large-scale structures in the cosmos.
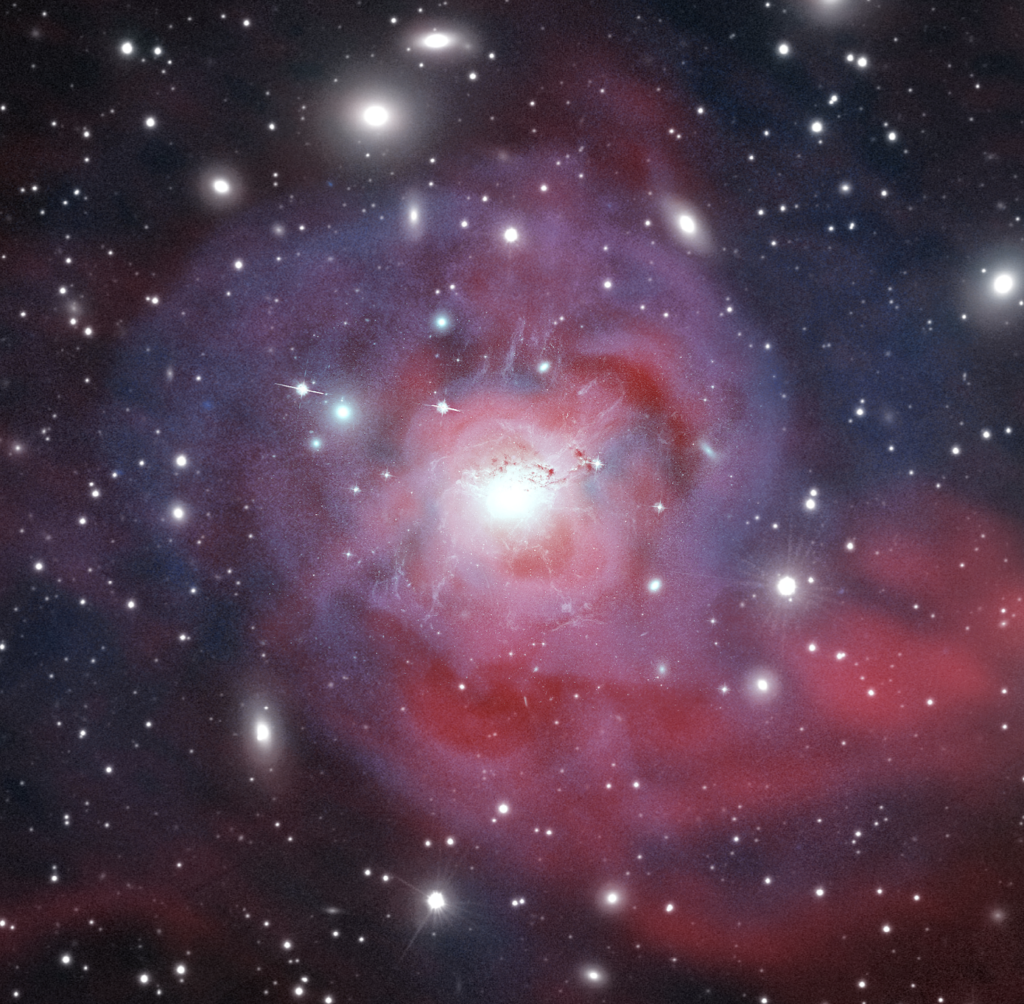
Deep Radio Observations: Shedding Light on the Hidden Universe
Through deep radio observations, our team is uncovering subtle structures within galaxy clusters that were previously unseen. These include mini-halos and phenomena such as Kelvin-Helmholtz instabilities, which provide valuable insights into the behavior of relativistic particles and magnetic fields. By producing detailed radio images, we contribute to advancing the field of radio astronomy and enhancing our understanding of the dynamic environments within clusters.
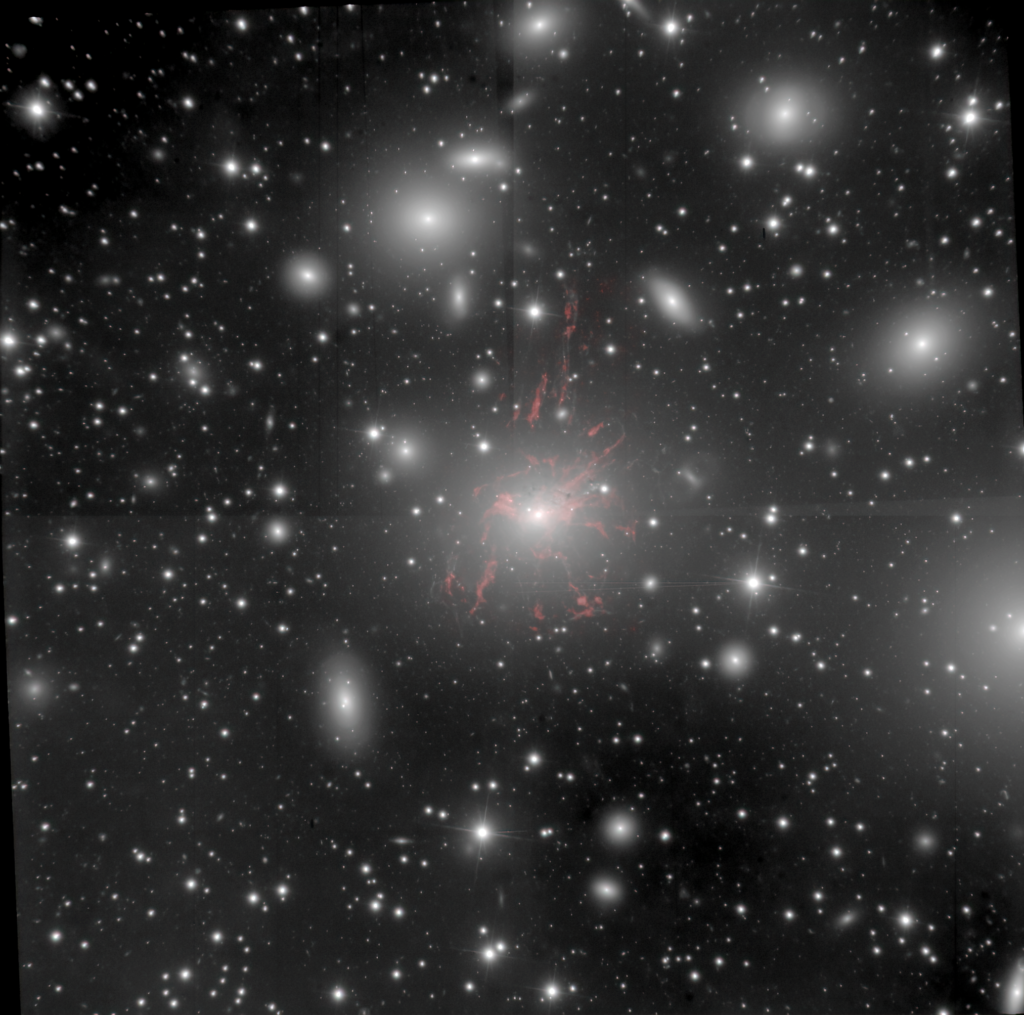
Multiphase Gas in Clusters: Balancing Cooling and Heating
We study the complex dynamics of cooling and heating in galaxy clusters, focusing on the interplay between hot and cold gas phases. Observations of systems like the Perseus cluster allow us to explore how black holes influence gas behavior and regulate star formation. By combining observational data with theoretical models, our work seeks to illuminate the processes that maintain thermal balance in these extreme environments.
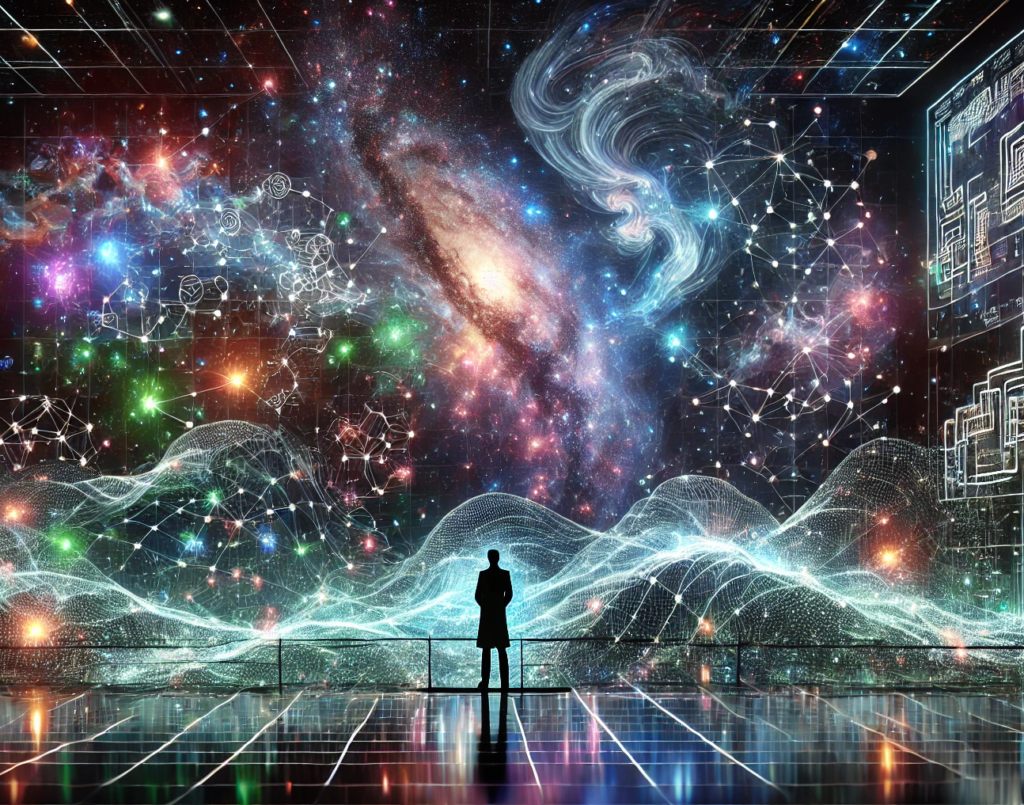
Machine Learning in Astrophysics: Enhancing Discovery
Our team is integrating novel machine learning techniques to address the challenges posed by large datasets from next-generation telescopes. By developing tools such as LUCI for integral field unit observations and applying neural networks to X-ray spectroscopy, we are improving data analysis efficiency and uncovering new possibilities for research. These efforts are helping to push the boundaries of what can be achieved with modern astrophysical datasets.